Note
Go to the end to download the full example code.
Using line search with PARAFAC
Example on how to use tensorly.decomposition.parafac
with line search to accelerate convergence.
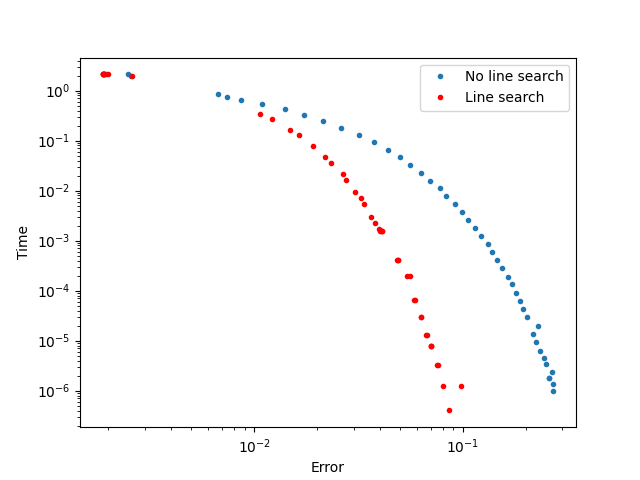
import matplotlib.pyplot as plt
from time import time
import numpy as np
import tensorly as tl
from tensorly.random import random_cp
from tensorly.decomposition import CP, parafac
tol = np.logspace(-1, -9)
err = np.empty_like(tol)
err_ls = np.empty_like(tol)
tt = np.empty_like(tol)
tt_ls = np.empty_like(tol)
tensor = random_cp((10, 10, 10), 3, random_state=1234, full=True)
# Get a high-accuracy decomposition for comparison
fac = parafac(tensor, rank=3, n_iter_max=2000000, tol=1.0e-15, linesearch=True)
err_min = tl.norm(tl.cp_to_tensor(fac) - tensor)
for ii, toll in enumerate(tol):
# Run PARAFAC decomposition without line search and time
start = time()
cp = CP(rank=3, n_iter_max=2000000, tol=toll, linesearch=False)
fac = cp.fit_transform(tensor)
tt[ii] = time() - start
err[ii] = tl.norm(tl.cp_to_tensor(fac) - tensor)
# Run PARAFAC decomposition with line search and time
for ii, toll in enumerate(tol):
start = time()
cp = CP(rank=3, n_iter_max=2000000, tol=toll, linesearch=True)
fac_ls = cp.fit_transform(tensor)
tt_ls[ii] = time() - start
# Calculate the error of both decompositions
err_ls[ii] = tl.norm(tl.cp_to_tensor(fac_ls) - tensor)
fig = plt.figure()
ax = fig.add_subplot(1, 1, 1)
ax.loglog(tt, err - err_min, ".", label="No line search")
ax.loglog(tt_ls, err_ls - err_min, ".r", label="Line search")
ax.legend()
ax.set_ylabel("Time")
ax.set_xlabel("Error")
plt.show()
Total running time of the script: (0 minutes 3.835 seconds)